Research Secondment in Local Area Energy Representation with Advanced Infrastructure
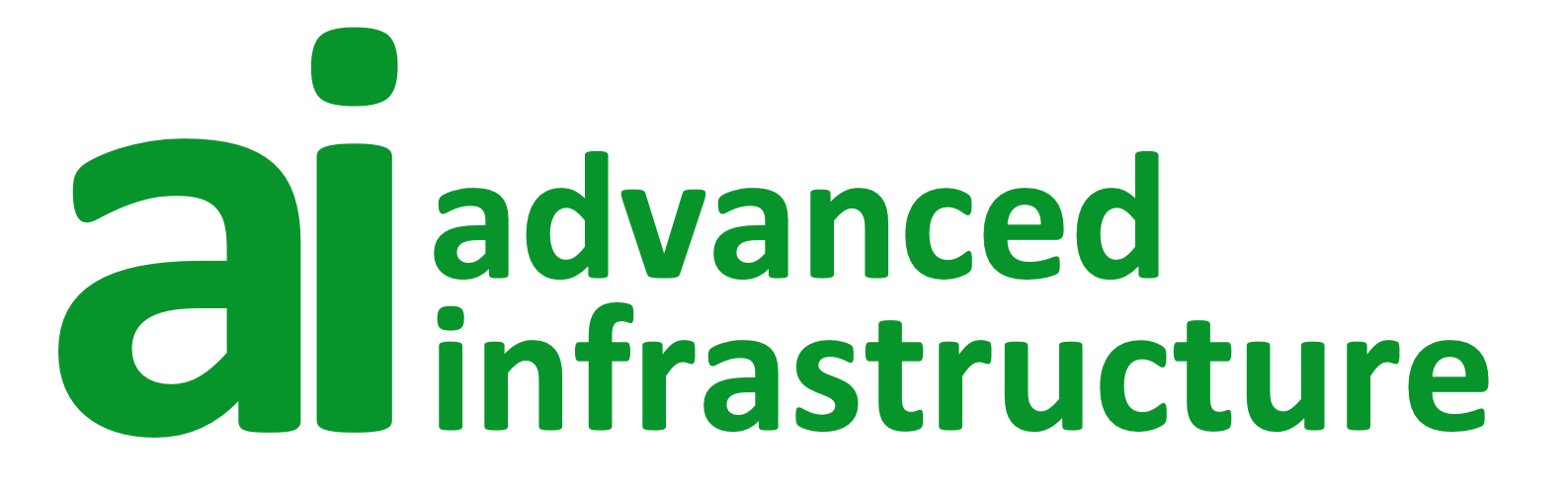
This post provides a new opportunity for a postdoctoral researcher from a C-DICE HEI to undertake a project with an industry sponsor related to net zero.
Industrial Sponsor: Advanced Infrastructure Technology
Advanced Infrastructure links energy and geospatial data to create powerful tools for low carbon infrastructure owners constructing smart grids. We use building and property data mapped to energy demand and appliance data to engineer building-stock demand profiles ten-times higher resolution than the industry standard.
The household is the level of granularity required for effective LCT deployments at a regional level. Local Energy Asset Representation (LEAR) is an emerging discipline that attempts to build city-level digital twins across the domains of heat, power, and transport, so that integrated Smart Local Energy Systems can be implemented. Current methods for LEAR creation require manual methods to identify infrastructure. Solutions that use automated techniques to infer energy asset characteristics would allow for national-scale digital twins to emerge. However, automated techniques would allow for faster and always-up-to-date digital twins. Methods to analyse and infer strategic decision making by energy network operators and relevant third parties in the public and private sectors, are required in order to increase the rate of deployment of LCTs. These methods should make use of newly available large datasets and cloud-based data architectures, for optimal strategic decision making.
Job Purpose
This research project will develop advanced data aggregation techniques for collating energy and energy-related data (including but not limited to: generation, consumption, demographic, building, weather, regulatory, and demographic data) from various sources, to build a complete profile of the UK energy system at the household, community, city, regional, and national level. These techniques will incorporate state-of-the-art uncertainty quantification and artificial intelligence techniques to account for expert knowledge and scarce data.
To meet 2050 net zero targets the UK needs to deploy smart grids at a rate of £20billion/year but is currently only reaching 10% of that target because of a bottleneck in data analytics for project planning. We link data between building stock and energy demand with the added value of high resolution demand forecasting for smart grid design. Competitors lack the modelling functionality required for distributed energy system modelling combined with high resolution geospatial and temporal demand profiles.
Special Conditions
This research secondment is open to postdoctoral researchers based at C-DICE HEIs, you will be required to seek permission from your employing organisation to undertake the secondment. The project will commence in Q1 2022.
All staff have a statutory responsibility to take reasonable care of themselves, others and the environment and to prevent harm by their acts or omissions. All staff are therefore required to adhere to the Advanced Infrastructure’s Health, Safety and Environmental Policy & Procedures.
All staff should hold a duty and commitment to observing the Advanced Infrastructure’s Equality, Diversity, and Inclusion policy and procedures at all times. Duties must be carried out in accordance with relevant Equality & Diversity legislation and Advanced Infrastructure’s policies/procedures.
Salary/FTE
Salary is University Pay Scale Spine 26 to 36 (£31,406 to £40,927 p.a. prorated for duration of post), depending on current postdoctoral researcher salary. Please note that reduced overheads will be paid due to the developmental opportunity this presents to the postdoctoral researcher to placed at Advanced Infrastructure.
We are open to this role being worked flexibly. The initial budget for this role is for 1.0 FTE for three months. However, we are open to this being worked flexibly, for example, working 0.5FTE for six months, as long as you are available for key meetings and activities, and outputs are delivered on time.
Location
The work may be done remotely or on the Advanced Infrastructure site in Cambridge.
Organisational Responsibility
Reports to: Christopher Jackson (Advanced Infrastructure Technology)
Person Specification
Essential Criteria
- Experience in Energy/Power Systems Modelling, specifically:
- Energy System Ontologies
- Energy System Modelling
- Distribution Network Power Flow Analysis
- Sincal/Matlab
- Uncertainty Propagation in Energy Systems Models
- Complexity Modelling
OR
*Experience in Building Engineering Physics, specifically: - Building Ontologies/Age/Building Standards
- Linking premises to physical buildings and type of activity
- Heating: Heat Pump and heating systems modelling
- Transport Modelling
- Writing and publication of reports, articles or papers
- Ability to undertake the duties and responsibilities of the post
- Excellent written and oral communication skills
- Excellent interpersonal and organisational skills
- Willingness to undertake appropriate further training and to adopt new procedures as and when required
- A good undergraduate degree.
- A PhD in an area related to one of the following: construction management, building technology, innovation, futures, management.
- Commitment to observing the Mace’s Equal Opportunities policy at all times
- Willingness to travel
Desirable Criteria
- Experience with Geospatial Analysis, specifically:
- Experience using GIS tools such as QGIS, ArcGIS (ESRI), CARTO and GIS Python libraries such as: Geopandas, GDAL, Ipyleaflet/Folium.
- Integrating GIS tools with multi-area energy systems
- Experience with Data Science, specifically:
- Python, in particular packages such as Pandas, Numpy, SciPy, PySpark, Tensorflow. A knowledge in Scala, Julia.
- Data cleaning
- Data aggregation
- Uncertainty characterisation and visualisation in complex data
- Machine learning (deep learning, clustering, probabilistic classification, time series forecasting)
- Gaussian Process Regression
Mentoring / Training Benefit to the Secondee
Designing for future significant nationwide scale-up is critical. The Secondee will be challenged with producing a solution which flourishes when widely deployed, enabling the range of services which can be provided to expand, whilst ensuring maintenance is low cost and effective. This is an emerging market with considerable uncertainty in the range of capabilities which will be required of the technical solution. The Associates, therefore, will need to embed flexibility into their work.
Modelling energy flows in buildings has been developed to a high level with sophisticated, detailed models for single buildings with multiple zones and complex servicing systems, including on-site generation. However, the level of input and detail required makes this approach impractical for large numbers of buildings using manual data input. Existing stock models are basic, typically using archetypes to represent many buildings scaled up to stock level, and make heroic assumptions about inputs in the absence of more data, such as using standard demand profiles for large numbers of, in reality, diverse buildings. There are numerous challenges such as the mapping of premises to buildings (one-one, one-many, many-one); understanding building usage to predict patterns of energy demand; limited knowledge of the thermal envelope and systems; and finally the sheer diversity of both domestic and non-domestic stocks.
Meanwhile, interaction between buildings and the electricity grid is of increasing importance due to decarbonisation of heat from gas to electric; significant on-site generation such as solar PV; battery storage; and an emerging electric vehicle fleet often connected to the same circuits as the building. These all interact to create net demand and are themselves evolving rapidly.
Creating datasets and models of building energy for local energy systems is an exciting academic challenge across several disciplines – building physics and services, stock data, data science, climate change (affecting future loads) etc. In this project, data from the power, heat, transport, sociodemographic, and geospatial domains, will be analysed across multiple dimensions and time series. At present, this task is performed mostly via unsophisticated methods and low spatial-temporal resolution analysis, resulting in flawed outcomes and sub-optimal decisions.
The aim would be to bridge the gap between the current single building approach, and the existing rather basic, ad hoc stock level methods. This is particularly important now more than ever, as buildings become increasingly active (i.e., possess energy generation capabilities) and smart. And what this development means for the electricity network is still not fully known. The outcome of this project could feed into new research projects, and has global relevance in research and teaching terms.
Eligibility
C-DICE target audiences are PD&ECRs as defined by “a person professionally conducting research after the completion of their doctoral studies and/or while undertaking research as a member of staff at a collaborating institution”. The PD&ECR must be currently employed by a C-DICE HEI (home institution) or partner.
This opportunity is not intended for permanent teaching staff / lecturers.
Timeline
• Deadline for applications: 24th November 2021 at 12:00
• Shortlisted applicants will be contacted within two weeks after the application deadline.
Submission Procedure
Applications may be submitted at any time prior to the deadline. Apply via Inkpath using Activity Code DSAB0002.